May 21-23, 2025
This workshop follows the prior Workshop on Analog physics-based computing in August of 2020, also sponsored by the Pittsburgh Quantum Institute. For the full videos of that meeting (all-online during Covid lockdown), see this link. The topics of that meeting have been expanded for the present meeting as described below. We expect this to be a lively meeting in which we discuss what can and cannot be done without a general-purpose quantum computer.
In addition, before the meeting, also in Pittsburgh, will be a Two-Day Tutorial on Tensor Network Methods on May 19-20, led by Juan José Mendoza Arenas of the University of Pittsburgh. More information on the tutorial is available here.
Afternoon excursion to Ohiopyle waterfalls will be provided for a fee of $40.
Topics of the Workshop:
Analog computational methods. It can be proven that no classical computer, whether digital or analog, can beat a fully quantum computer in the limit of N→∞, where N is the number of processed bits. However, as extensively discussed at the 2020 workshop, an analog system can potentially rival a quantum computer on NP-hard problems when N is large but not infinite.
Quantum simulators. These systems are defined as ones in which quantum mechanics plays a crucial role, such that they cannot be described as classical, but they are designed to solve just one class of problems instead of being a general quantum computer.
Quantum-inspired digital algorithms. Many classes of problems that relate to the real world involve a truncation of the whole information of a many-body wave function. Therefore it can be possible to use classical digital computers to solve some of the same problems originally addressed by quantum computing algorithms.
Registration:
The registration form for this workshop is available above. Registration is free for students and faculty of PQI-affiliated institutions. Registration for others is $100 for students and $250 for senior researchers. There is no extra charge for the Tutorial on May 19 & 20.
Travel arrangements:
Attendees traveling to Pittsburgh by commercial air flight should book to Pittsburgh International Airport (PIT). There is an inexpensive direct city bus line to the Oakland section of Pittsburgh, where the meeting will be held, which takes about 45 minutes. Alternatively, Uber or Lyft cost about $50 and take about 30 minutes to Oakland from the airport, depending on traffic.
The venue will be the Cathedral of Learning of the University of Pittsburgh. Hotels nearest to the venue are the Mariott Courtyard University Center, the Oaklander Hotel, and the Hilton Garden Inn University Place
Organizing committee:
- David Snoke, University of Pittsburgh (Chair)
- Natalia Berloff, University of Cambridge
- Pedram Khalili, Northwestern University
- Vincent Liu, University of Pittsburgh
- Juan José Mendoza Arenas, University of Pittsburgh
Schedule:
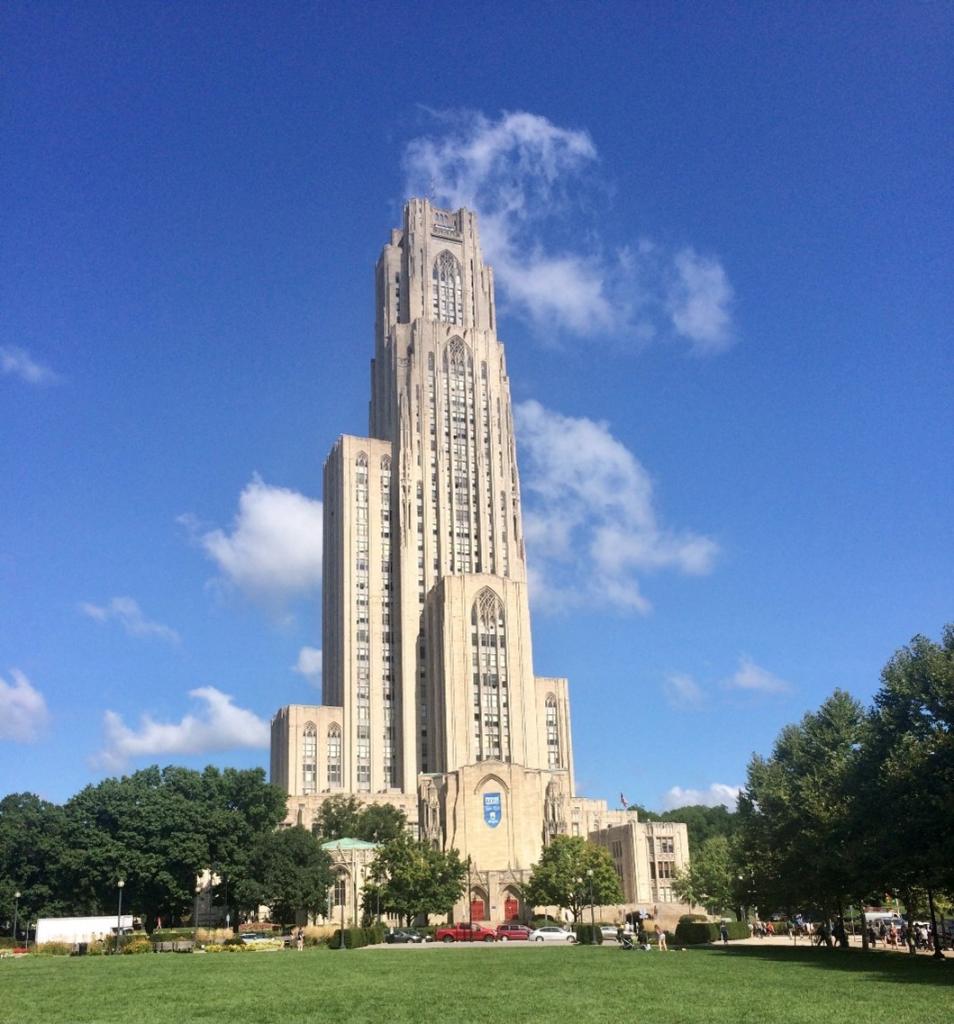
WEDNESDAY, May 21
9:30 AM - “A tensor network perspective on quantum computing and quantum simulation”
Miles Stoudenmire, Flatiron Institute, New York, USA
Many quantum systems have efficiently computable properties, despite an exponential worst-case scaling. This is because quantum systems exhibit a wide range of structures, including weak correlation, scale separation, limited entanglement, and locality. Developments in classical algorithms based on tensor networks put increasingly strong constraints on the kinds of problems that require a quantum computer, since tensor networks can perform many of the same tasks while also allowing efficient operations that are inefficient to perform with quantum hardware. I will discuss the implications of tensor network developments for a range of envisioned quantum computing algorithms and applications, including the quantum Fourier transform, Grover's algorithm, and simulation of quantum dynamics.
10:15 AM - “Simulated bifurcation: a quantum-inspired highly-parallelizable algorithm for combinatorial optimization”
Kosuke Tatsumura, Toshiba Corporation, Japan
Judging, decision-making and/or planning based on analyzing many correlated factors are often mathematically formulated to a quadratic discrete optimization problem known to be a non-deterministic polynomial-time hard (NP-hard) problem. Simulated bifurcation (SB) is a quantum-inspired heuristic algorithm for quadratic discrete optimization and finds the exact or approximate ground state of the Ising spin model onto which the target problem is mapped. The SB algorithm was found as a classical counterpart of bifurcation-based adiabatic quantum computation with a nonlinear oscillator network. In SB, we numerically simulate the adiabatic evolution of a classical Hamiltonian system (a nonlinear oscillator network) with bifurcations (Fig. 1). Two branches of a bifurcation in each nonlinear oscillator represent two states of each Ising spin. The operational mechanism of SB is based on an adiabatic and ergodic (/probabilistic) search.
The SB algorithm is highly parallelizable and can be accelerated with massively parallel processors such as FPGAs and GPUs. The highly parallel implementations of SB, called simulated bifurcation machines (SBMs, one of the Ising machines), have been demonstrated to be competitive with the other state-of-the-art Ising machines. FPGA-based SBMs are applicable/suitable to high-speed real-time systems [3-6] because they can be embedded together with other system components (machine learning, communication, data buffer, judgment, etc.) in the FPGA to shorten the system-wide latency. Real-time systems are defined as those systems that must respond (sense, judge, and react) within critically defined time constraints. In this presentation, we will review our recent works on simulated bifurcation machines with emphasis on their applications to real-time systems that make NP-hard optimization-based (rational) responses to rapidly changing situations.
11:00 AM - Coffee break
11:20 AM - “Quantum-inspired simulations of fluid dynamics”
Juan Jose Mendoza Arenas, Swanson School of Engineering, University of Pittsburgh, USA
The matrix product state (MPS) representation, developed for approximating the state of quantum many-body systems, exploits their correlation structure to accurately capture the underlying physics in a low-rank form (i.e., in a massively reduced state space). Here, the methodology is employed for simulating compressible and chemically reacting flows. In doing so, the governing differential operators of the underlying Navier-Stokes equations are recast in the context of MPS, and their dynamics is simulated with various degrees of truncation. Simulations are performed to assess the effects of the Reynolds number, the Mach number and chemical heat release ratios on the compositional structure of the flow. The results via MPS-reduced order solutions are appraised against those generated via direct numerical simulation of the same flows.
11:55 AM - “Quantum simulation with higher-dimensional tensor networks”
Joey Tindall, Flatiron Institute, New York, USA
In this talk I will present very recent results and advances from using two and three-dimensional tensor networks for quantum simulation. I will first discuss our graphical approach to working with these complex mathematical objects which, when combined with belief-propagation-based contraction schemes, lets us efficiently optimize and contract tensor networks of generic structure to high accuracy. I will also introduce our open-sourced codebase ITensorNetworks.jl, an extension of the ITensors.jl library, where these routines are implemented and usable.
I will then focus on a recent quantum supremacy experiment from DWave, where the dynamics of a quantum annealing protocol on a range of lattices was claimed to be beyond the reach of classical resources. I will show that this is not the case and, for fixed annealing time, classical two-and-three-dimensional tensor network simulations can be performed which are more accurate than the Quantum Processing Unit and scale only linearly in the number of qubits. I will then show how our tensor network approach can capture the universal physics present in these dynamic simulations by performing a scaling collapse of the correlation function and extracting the Kibble-Zurek exponent.
I will conclude by discussing the generality of our results to future simulations, with complex two and three-dimensional quantum circuits and quantum dynamics problems now becoming accessible to tensor network methods.
12:30 PM - Lunch
2:00 PM - “Pushing the boundary of quantum advantage in hard combinatorial optimization with probabilistic computers”
Shuvro Chowdhury, OPUS Lab, University of California at Santa Barbara, USA
Recent demonstrations on specialized benchmarks have reignited excitement for quantum computers, yet whether they can deliver an advantage for practical real-world problems remains an open question. Here, we show that probabilistic computers (p-computers) when co-designed with hardware to implement powerful Monte Carlo algorithms can surpass state-of-the-art quantum annealers in solving certain hard optimization problems. We focus on two key algorithms: discrete-time simulated quantum annealing (DT-SQA) and adaptive parallel tempering (APT), both applied to 3D spin glasses. For DT-SQA, we find that increasing the number of replicas improves residual energy scaling, while parallelizing fewer replicas across independent runs also achieves comparable scaling. Both strategies align with the theoretical expectations from extreme value theory. In addition, APT outperforms DT-SQA when supported by non-local isoenergetic cluster moves. Finite-size scaling analysis suggests a universal behavior that explains the superior performance of APT over both DT-SQA and quantum annealing. We show that these algorithms are readily implementable in modern hardware thanks to the mature semiconductor technology. Unlike software simulations, replicas can be monolithically housed on a single chip and a large number of spins can be updated in parallel and asynchronously, similar to a quantum annealer. We project that custom Field Programmable Gate Arrays (FPGA) or specialized chips leveraging massive parallelism can further accelerate these algorithms by orders of magnitude, while drastically improving energy efficiency. Our results raise the bar for a practical quantum advantage in optimization and present p-computers as scalable, energy-efficient hardware for real-world optimization problems.
2:45 PM - “Ultracold atoms in optical lattices: quantum simulators for many-body physics”
Ulrich Schneider, University of Cambridge, UK
Ultracold atoms in optical lattices are one of the major platforms for experimental quantum simulations and many-body physics. Their versatile nature and high controllability enable the realization of novel quantum phases and the study of phase transitions and out-of-equilibrium dynamics.
Optical lattices intrinsically combine large coherence times and system sizes significantly beyond what can be simulated classically. In the past, they were however often limited to simple lattice geometries, namely the primitive 1D lattice, the square lattice, and the cubic lattice, and were mostly geared towards translation-invariant systems.
In this talk, I will present several current experimental developments to extend these platforms to additional 2D lattice geometries and to add local programmability to for example address larger classes of many-body problems.
3:30 PM - Coffee break
4:00 PM - Panel/Open Discussion
“Optimization is more important technologically than Shor’s algorithm. What is the best way forward?”
THURSDAY, MAY 22
9:30 AM - “From Stuart-Landau dynamics to XY minimization: Physical oscillators for phase retrieval and beyond”
Natalia Berloff, Department of Applied Mathematics and Theoretical Physics, University of Cambridge, UK
Recent advances in non-equilibrium physical platforms—from gain-dissipative photonic networks to condensate-based oscillators—have underscored their natural ability to emulate Stuart-Landau-type dynamics and minimize continuous-variable XY Hamiltonians. These systems simultaneously permit amplitude and phase evolution, offering high-dimensional search capabilities well-suited for complex optimization tasks. In particular, we show how they effectively map the Coded Diffraction Pattern (CDP) phase retrieval problem onto an XY minimization framework, outperforming conventional iterative algorithms in both accuracy and noise robustness. Beyond phase retrieval, similar principles can guide the solution of other optimization problems, including sensor placement, indirect correlations detection and quadratic binary optimization problems. In this talk, I will highlight the core theoretical underpinnings connecting Stuart-Landau dynamics, XY Hamiltonians, phase retrieval and other optimization tasks, then present strategies to enhance the convergence of such physical solvers toward true global minima.
10:15 AM - “Solving computational problems with coupled lasers”
Nir Davidson, Weizmann Institute of Science, Rehovot, Israel
Computational problems may be solved by realizing physics systems that can simulate them. Here we present a new system of coupled lasers in a modified degenerate cavity that is used to solve difficult computational tasks. The degenerate cavity possesses a huge number of degrees of freedom (300,000 modes in our system), that can be coupled and controlled with direct access to both the x-space and k-space components of the lasing mode. Placing constraints on these components are mapped on different computational minimization problems. Due to mode competition, the lasers select the mode with minimal loss to find the solution. We demonstrate this ability for simulating XY spin systems and finding their ground state, for phase retrieval, for imaging through scattering medium, and more.
11:00 AM - Coffee break
11:20 AM - “Polariton BEC in lattices: Introduction and open questions”
David Snoke, Department of Physics and Astronomy, University of Pittsburgh, USA
I will give a basic introduction to polariton superfluids in lattices, and discuss the question of the time scale for condensation into the ground state, including results from recent experiments measuring the coherence properties of a polariton condensate. The question of time scale for condensation is paramount for whether polariton superfluids can scale to large numbers of lattice sites to solve NP-hard problems.
11:55 AM - “Orbital optical lattices: from topological Bose-Einstein condensation to gravitational wave detection”
W. Vincent Liu, Department of Physics and Astronomy, University of Pittsburgh, USA
Orbital is a fascinating degree of freedom independent of charge and spin. It is known to play a rudimentary role in understanding the nature of superfluid pairings, such as s-, p-, and d-wave. In condensed matter, through its coupling to spin and charge, orbital order intertwines superconductivity, magnetism, and other quantum phenomena. The advanced spatiotemporal control of cold atoms provides new opportunities to explore orbital physics beyond standard quantum regimes, complementary to studies in condensed matter. In this talk, I will report on theoretical and experimental progress in orbital superfluidity, highlighting unique phases of matter emerging from the interplay of novel spatial lattice geometry and orbital symmetries. Notable examples include chiral p-wave Bose-Einstein condensates with topological excitations and a proposal to use the squeezed (px, py) orbital states as a pseudospin for detecting gravitational waves and dark matter.
12:30 - Excursion to Ohiopyle waterfalls and trail biking
FRIDAY, MAY 23
9:30 AM - “The many faces of optical neural networks”
Alexander Lvovsky, Clarendon Laboratory, University of Oxford, UK
Optical neural networks (ONNs) harness the fundamental properties of light to enable ultrafast, energy-efficient computation, surpassing the limitations of digital-electronic systems in tasks such as large-scale matrix multiplications. By exploiting interference, diffraction, and nonlinearity, ONNs can perform parallel processing of high-dimensional data, reducing latency and power consumption. This talk explores three complementary perspectives on ONNs. First, we discuss ONN applications for machine learning and our recent results on training an ONN by propagating light backwards through its layers. Second, we show how an ONN can be applied for spatial mode decomposition of an optical field, enabling the extraction of spatial information beyond the classical diffraction limit by leveraging the quantum and classical correlations in the field. Finally, we discuss coherent Ising machines, in which an optical network with feedback finds minimum-energy gates of interacting spin systems to solve nontrivial combinatorial optimization problems. In particular, we highlight our recent findings on polarization symmetry breaking, offering a new mechanism for all-optical implementation of an Ising machine. Together, these facets illustrate the versatility and transformative potential of ONNs.
10:15 AM - “Coherent Ising machine based on network of optical parametric oscillators”
Hiroki Takesue, NTT Basic Research Laboratories, Japan
As the development of digital computers nears saturation, there is increasing research and development focused on creating computers that efficiently solve problems that traditional digital computers find challenging, using the dynamics of physical systems. One notable approach is the “Ising machine,” which converts combinatorial optimization problems into the task of finding the ground state of the Ising model, solving these problems through experiments with artificial spin systems. NTT is working on a coherent Ising machine (CIM) that simulates the Ising model using coupled degenerate optical parametric oscillators (DOPO) as an artificial spin system. In this presentation, I will discuss the principles of CIM, the current status of the large-scale CIM developed at NTT, and its applications to combinatorial optimization problems, as well as future developments.
11:00 AM - Coffee break
11:20 AM - “Scalable architectures for photonic compute-in-memory using nonvolatile optical materials”
Nathan Youngblood, Swanson School of Engineering, University of Pittsburgh, USA
Photonics information processing strategies offer the unique ability to perform analog computation with ultra-low latency and high efficiency. However, designing compact and reconfigurable photonic architectures which scale well is a challenge. The combination of bistable optical materials (such as phase-change materials like Ge2Sb2Te5) and integrated photonics is a promising approach which enables nonvolatile optical memory on-chip with low drift, compact footprint, and high-speed readout. This talk will focus on developing robust and scalable photonic memories using phase-change and magneto-optic materials (Ce:YIG)—together with wavelength division multiplexing and “in-memory” computing techniques—to enable high-speed matrix-vector operations for machine learning applications.
11:55 PM - Integrated probabilistic computer using voltage-controlled magnetic tunnel junctions as its entropy source
Christian Duffee, Jordan Athas, and Pedram Khalili Amiri, Department of Electrical and Computer Engineering, Northwestern University
Probabilistic Ising machines (PIMs) provide a path to solving many computationally hard problems more efficiently than deterministic algorithms on von Neumann computers. Stochastic magnetic tunnel junctions (S-MTJs), which are engineered to be thermally unstable, show promise as entropy sources in PIMs. However, scaling up S-MTJ-PIMs is challenging, as it requires fine control of a small magnetic energy barrier and duplication of area-intensive digital-to-analog converter (DAC) elements across large numbers of devices. In addition, non-spintronic components of S-MTJ-PIMs to date have been primarily realized using general-purpose processors or field-programmable gate arrays. Reaching the ultimate performance of spintronic PIMs, however, requires co-designed application-specific integrated circuits (ASICs), combining CMOS with spintronic entropy sources. Here we demonstrate an ASIC in 130 nm foundry CMOS, which implements integer factorization as a representative hard optimization problem, using PIM-based invertible logic gates realized with 1143 probabilistic bits. This experimental setup is shown in Fig. 1. The ASIC uses stochastic bit sequences read from an adjacent voltage-controlled (V-) MTJ chip. The V-MTJs are designed to be thermally stable in the absence of voltage and synchronously generate random bits without the use of DAC elements using the voltage-controlled magnetic anisotropy effect. We experimentally demonstrate the chip’s functionality and provide projections for designs in advanced nodes, illustrating a path to millions of probabilistic bits on a single CMOS+V-MTJ chip.
12:30 PM - Lunch
2:00 PM - Ising Machines Based on Spin Hall Nano-Oscillators, Spin Waves, and Acoustic Waves
J. Åkerman, A. Litvinenko, A. Kumar, A. A. Awad, R. Khymyn, R. Ovcharov, and V. H. Gonzalez
Department of Physics, University of Gothenburg, Sweden
Ising machines (IMs) solve combinatorial optimization problems heuristically by minimizing the energy of the Ising Hamiltonian the problem maps to. IMs can be realized in different types of hardware, some based on spatial networks, such as D-Wave’s quantum annealer, and others based on a time-multiplexed approach, such as the optical Coherent Ising Machine (CIM). While the time-multiplexed CIM realizes an all-to-all IM, circumventing spatial IMs’ scaling and connectivity problems, they do not offer an easy route for miniaturization, requiring kilometer-long optical fibers and non-linear optical elements. In this talk, we present our recently demonstrated spin-wave-based time-multiplexed IM (SWIM) using mm-long YIG films and our fully FPGA-programmable time-multiplexed IM based on surface acoustic waves (SAWs). We also present our recent research towards spatial IMs based on large arrays of spin Hall nano-oscillators (SHNOs).
2:45 PM - “Impact of random bitstream quality on probabilistic Ising machines using CMOS and voltage-controlled magnetic tunnel junctions”
Jordan Athas, C. Duffee, and Pedram Khalili Amiri, Department of Electrical and Computer Engineering, Northwestern University, USA
The performance of probabilistic Ising machines is closely tied to the quality of their entropy (i.e., randomness) source, which influences their ability to explore solution spaces effectively. In this work, we investigate how hardware-generated randomness affects the solution process of Ising optimization problems, particularly the Max-Cut problem. While quantum computing leverages intrinsic quantum randomness, we explore how classical hardware-based randomness can approximate similar effects in digital Ising solvers.
We develop a true random number generator (TRNG) using voltage-controlled magnetic tunnel junction (VC-MTJ) as an entropy source, leveraging its ability to generate random bits in a field-free configuration. This enables seamless CMOS integration while reduction system complexity. However, fabrication variation and environmental factors introduce bias in the bitstream, necessitating post-processing [1]. To refine the entropy quality, we integrate the VC-MTJ with CMOS-based linear feedback shift registers (LFSRs) and exclusive-OR (XOR) gates [2]. A version of the design with four LFSRs is presented in Figure 1. This hybrid design balances true randomness with pseudo-random augmentation, improving the statistical uniformity of the generated bitstream.
We evaluate the impact of different entropy sources—including raw VC-MTJ output and increasingly complex VC-MTJ + LFSR configurations—on the performance of a digital Ising machine solving various Max-Cut problems. These bitstreams serve as inputs to an Ising machine tasked with solving the Max-Cut problem. By analyzing solution accuracy, time-to-solution, and optimal temperature settings, we assess how well these quantum-inspired randomness sources enhance probabilistic Ising solvers. Our findings provide insights into optimizing entropy sources for scalable and efficient classical Ising-based computing, bridging the gap between quantum and classical approaches to combinatorial optimization.
3:20 PM - Coffee break
3:40 PM - “GroverGPT”
Junyu Liu, School of Computing and Information, University of Pittsburgh, USA
Quantum computing is an exciting non-Von Neumann paradigm, offering provable speedups over classical computing for specific problems. However, the practical limits of classical simulatability for quantum circuits remain unclear, especially with current noisy quantum devices. In this work, we explore the potential of leveraging Large Language Models (LLMs) to simulate the output of a quantum Turing machine using Grover’s quantum circuits, known to provide quadratic speedups over classical counterparts. To this end, we developed GroverGPT, a specialized model based on LLaMA’s 8-billion-parameter architecture, trained on over 15 trillion tokens. Unlike brute-force state-vector simulations, which demand substantial computational resources, GroverGPT employs pattern recognition, reinforcement learning and Chain-of-Thought Prompting (CoT) to approximate quantum search algorithms without explicitly representing quantum states. Analyzing 97K quantum search instances, GroverGPT consistently outperformed OpenAI’s GPT-4o (45% accuracy), achieving nearly 100% accuracy on 6- and 10-qubit datasets when trained on 4-qubit or larger datasets. It also demonstrated strong generalization, surpassing 95% accuracy for systems with over 20 qubits when trained on 3- to 6-qubit data. Analysis indicates GroverGPT captures quantum features of Grover’s search rather than classical patterns, supported by novel prompting strategies to enhance performance. Although accuracy declines with increasing system size, these findings offer insights into the practical boundaries of classical simulatability. This work suggests task-specific LLMs can surpass general-purpose models like GPT-4o in quantum algorithm learning and serve as powerful tools for advancing quantum research and education.
4:15 PM - Reformulations and Decomposition for Quantum Discrete Optimization
David E. Bernal, Davidson School of Chemical Engineering, Purdue University
Quantum computing has the potential to accelerate specific challenging computational tasks compared to classical computers. In particular, given the Ising spin model to quadratic unconstrained binary optimization (QUBO) equivalence, methods have been proposed to tackle discrete optimization through quantum methods. We present a series of tools that allow reformulating discrete nonlinear optimization problems into QUBO and solving them using current quantum computers. Details can be found in https://arxiv.org/abs/2307.02577 and https://github.com/JuliaQUBO/QUBO.jl
We have explored several solution methods for those QUBO problems: quantum annealers, quantum entropic computers, classical methods such as parallel tempering and simulated annealing, and simulation of quantum approaches such as coherent Ising machines.
Our Tensor network solver, TenSolver, maps the QUBO instance to a Hamiltonian that can be approximated by a tensor network on its own. The bond dimension of the tensor network can be used to control the level of approximation, which also strongly affects its solvability. In particular, we use well-developed methods to contract tensor networks using GPUs, some of them implemented in the software iTensor or the library CUDA-TN, to reach scales of practical interest. We have developed a fully open-source implementation of our solver in the Julia language, in a package called TenSolver.jl, which can be found in the repository https://github.com/SECQUOIA/TenSolver.jl.