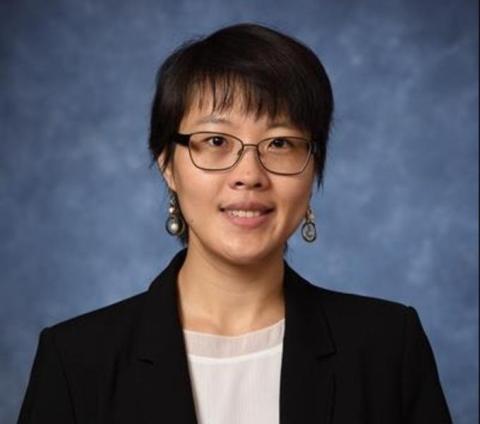
As additive manufacturing becomes more commonplace, researchers are exploring new methods to ensure that products are as strong and more sustainable than their traditionally manufactured analogues. At the University of Pittsburgh, engineers have set their sights on using multiple lights and neural networks to improve the finishing process of this complex process.
Xiayun Zhao, assistant professor of mechanical engineering and materials science at Pitt’s Swanson School of Engineering, received a Faculty Early Career Development (CAREER) award from the National Science Foundation (NSF) for her research in photopolymer additive manufacturing (PAM). This technology utilizes two lights to cure photosensitive materials and curb the associated overcuring, respectively, allowing for the fabrication of components with higher resolution and greater precision than existing PAM technologies.
Despite its potential, PAM still suffers from the intractable issue of overcuring, which can harm the structural integrity of printed parts. The $657,610, five-year award will support Zhao in developing a smart, novel digital light processing (DLP) method that capitalizes on photoinhibition induced by a 2nd wavelength light to curb curing parts (DLP2Curb). This process will enable researchers to manipulate cure and curb exposures of the PAM processing to improve the precision and strength of printed parts. The proposed cyber-physical system of DLP2Curb will facilitate the realization of Rapid, Intelligent, Precise, Extensive, Sustainable, and Transformative (RIPEST) PAM—that will enhance the geometrical fidelity and functional integrity of printed parts.
“Usually, we use a single-wavelength light to cure the polymer, but this new method will use two wavelengths—one to cure the material, and one to prevent the material from over-expanding into the surrounding area,” explained Zhao. “This way, we can increase both the accuracy and the resolution in three dimensions, and we can not only improve printing speed but also optimize both the lateral and vertical profiles of printed material."
If successful, this research will have a significant impact on a range of applications, including biochips, electrodes, soft robots, metamaterials, and others that require precision manufacturing of parts with complex shapes and strengthened performance.
There are three main phases to Zhao’s research. In the first, she and her team will try to understand how the DLP2Curb process works and how different materials behave under these conditions. This work will involve creating a digital twin—a complex computer model that will simulate how tweaks made to the process or material will change the outcome. In the second part, she will use tools like interferometric and ultrasonic monitoring systems to watch the materials in real-time to characterize PAM. The third and final part, Zhao will develop a way to control the process in real time, using deep reinforcement learning of model predictions and in-situ measurements to guide the printing process.
“Using a single wavelength to cure polymers while printing is already complex. Adding a second escalates that complexity, but we can use machine learning to enhance our accuracy and accelerate our progress,” explained Zhao. “Conventional machine learning calculations take time and aren’t fast enough for real-time control yet, but the digital twin we’ll develop as part of this project based on the state-of-the-art physics-informed neural networks will help us in that endeavor.”
In addition to the scientific contributions to the manufacturing industry, Zhao’s CAREER award will also provide educational activities aimed at training future leaders to pursue manufacturing-related careers and diversifying STEM workforce. As one of her extensive outreach program, Zhao plans to partner with the Carnegie Science Center to host summer camps that will teach students about AI and metrology-aided additive manufacturing and give them hands-on experience with 3D-printing for manufacturing.
From Pitt SSOE